Data paradise factory floor: get more out of your machine and production information
Közzétéve: 2020. szeptember 25. hely: AI
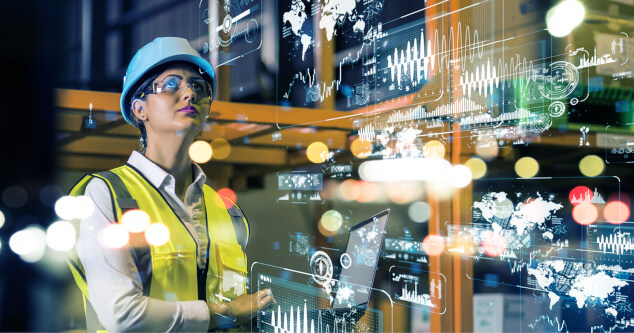
Data Science project approach: preparation, analysis and application development, evaluation and maintenance
Industrial Data Science is quite a new discipline. That’s why, there is (still) no generally valid approach that is suitable for every company. Every solution and application require customized data analysis and modelling to achieve the best possible result. However, a standard approach is useful. The CRISP-DM model, (Cross-Industry Standard Process for Data Mining) is the most commonly adapted basis. OMRON simplified and tailored CRISP-DM into a new approach. The four steps of this approach are preparation, analysis and application development, evaluation and maintenance.Phase 1: Preparation
The preparation phase is the most important phase. A data science project will never be successful if the goal is unclear. Therefore, in this first important step, all participants and area experts first deal with the problem or the specific requirement in order to arrive at a clearly defined project goal. They analyse the machine and/or the production process in detail in order to get an overview of which data is already available and which still needs to be collected. In this process, an initial data set can be collected and analysed as a kind of feasibility study. At the end of the preparation phase, a report is produced that provides insights into the expected generated value and a realistic ROI.Phase 2: Analysis and application development
- Data collection: Data is collected from various sources – from raw sensor data to information from MES systems.
- Data pre-processing: The collected data is prepared for the analysis step, transformed, merged and cleaned up.
- Data analytics: The developed analysis algorithms and machine learning models are applied.
- Application: The results and conclusions of the data analysis are made available. Examples are visualizations, tailored to the situation, target group or as feedback to the machine.